A Conversion Conversation with Online Dialogue’s Ton Wesseling
We often want to understand the long-term impact of a successful Experiment, but there are many pitfalls in doing so. I recently spoke to Ton about some of the factors to account for, as well as his passion for evidence-based work, and his take on running many experiments at once. Ton’s got a ton of experience and material to share. And he may have the most awesome caricature I’ve ever seen.
Rommil: Hi Ton, how are you? Thanks for taking the time to chat with me! Let’s kick things off with you sharing a bit about yourself and what you do?
Ton: My name is Ton Wesseling. You probably know me from keynotes, workshops, training or articles about conversion optimization and A/B-testing in particular. I’m the founder and owner of CRO Agency OnlineDialogue.com, the free ABtestguide.com toolset and the Conference formerly known as ConversionHotel.com
I’m a creator who loves data and strategy. I was born and raised in the Netherlands 45 years ago and I grew up as a kid in the 80’s with the first personal computers. During my time at university in the 90’s I fell in love with the web. A truly unique place to create, share, learn and optimize. I created my first websites back in those days and ended up as one of the first employees at an internet publishing start-up in the late 90’s.
I never left the internet industry. Over the last 22 years, my work shifted at first from creating to optimizing digital products and later on to creating and optimizing the process & structure within companies to deliver and maintain great digital products, services and shops. My journey went from applying evidence-based growth to embedding evidence-based growth.
That’s very interesting. I feel a lot of people in our field also took a similar path, myself included. How are you liking it?
I love this industry and the people in this field of work. It’s all based on having a growth mindset, which also motivates me a lot to keep on going forward. I still consult and teach and keep on publishing content and organizing events around evidence-based decision making and setting up and maintaining a structure and a culture of experimentation and validation.
So, what motivated you to found Online Dialogue and how is it different from other CRO agencies?
I founded Online Dialogue in 2009. I was amazed back in those days by the low quality of digital presence from the major brands and companies. Their digital efforts were run by redesigns, gut feel and were a combination of broken links, fascinating usability and terrible user dialogues.
We have data, we can run experiments! Those companies could be so much more effective, create less waste. Besides the direct business ROI: wouldn’t it be so much more fun to work on this all together in small sprints prioritized on evidence? Wouldn’t it be really rewarding to learn and understand what works and what doesn’t?
You would think. But it seems many places only look for evidence to prove there is a need, not necessarily evidence to prove their solutions work. Go on.
I convinced Bart Schutz to join me as a business partner. I worked with Bart on other projects before and he shared my annoyance on the quality of websites. Bart his background is consumer psychology and he was applying his knowledge on websites mostly without A/B-testing. I was A/B-testing a lot but I did not know anything about consumer psychology.
A match made in heaven, we started to show the world that data + psy = growth. That high quality researched hypothesis combined with trustworthy experimentation leads to really valuable insights in user behaviour. Insights to be monetized in the short-term and long-term. We had lots of fun, started to hire a team and won several awards in the early days of experimentation based web optimization. Our company grew and became our vehicle to prove to businesses that they should embrace validation in their organization, which is also our mission statement.
“data + psy = growth”
This whole background is also what differs Online Dialogue from other CRO agencies. We have a way above average knowledge of online user behaviour from a consumer psychology perspective, which grew with the thousands of experiments we performed over the last 10 years. This gives us a better ROI in conversion optimization projects we run.
What also differs us is a thorough knowledge of the methodology and statistics behind experimentation. Sadly I see many agencies not delivering trustworthy experimentation results and making bad decisions for their clients based on suboptimal approaches and wrong statistical assumptions.
Last but not least our company started consulting on and helping with building up inhouse experimentation programs and teams already several years ago, which currently makes 50% of our business. As consultants, we’ve learned through those years about the nitty-gritty details of maturity stages growing from a single optimization team to a center of excellence enabling experimentation and validation throughout the organization. This knowledge is also what differs us from other CRO agencies, who mostly focus on outsourced agency work.
As I was trying to figure out a topic for us to chat about, I ran across this very interesting LinkedIn post of yours:
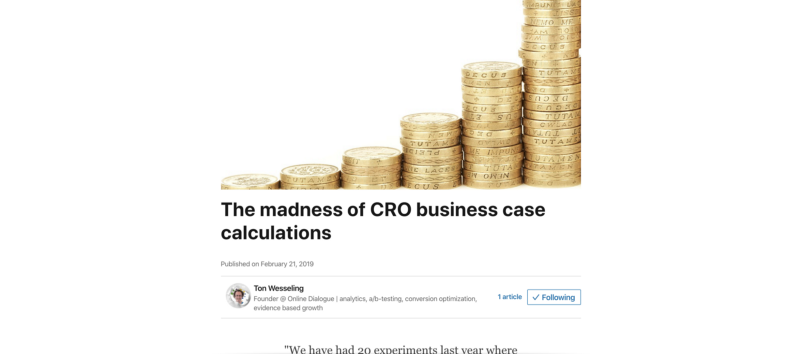
Could you share your thoughts about the impact of “False Detection Rate” on calculating the impact of a CRO program?
The main lesson of this article is that you should not calculate the potential financial impact of just 1 A/B-test. You need a group of A/B-tests to be able to do this. The result of an experiment is significant or not, which just means implement or not (in case you are looking for uplifts). The potential financial impact only happens if the measured result is true.
“…you should not calculate the potential financial impact of just 1 A/B-test. You need a group of A/B-tests to be able to do this.”
It’s even worse. Your measured outcome has a Magnitude Error (Type-M error), which means your measured difference in conversions is right-skewed towards the real difference. This is caused by the fact that the average conversion rate of all significant measured results of one experiment (if you repeat this many times) is higher than the real conversion rate because sometimes the measured result will be non-significant and that one doesn’t influence your average measured significant result.
But back to calculating the impact of your whole program, the impact of a group of experiments. What most testers don’t know is that they are probably running a program where only 50% of their winning outcomes of A/B-tests are real wins. This is why you should calculate your False Discovery Rate of your A/B-testing program. It could be your ROI is only 50% of what you thought it was….
So that’s very interesting. Let’s take this scenario. An Experimentation program has run, say, 100 tests on the same property, all trying to impact sales. How would you suggest predicting the contribution of Experimentation to revenue in this case?
Let’s say you’ve run those 100 experiments on a 90% significance level (this or higher in the measured outcome is seen as a win). We all know that with running 100 A/A-experiments (no difference between default and challenger) we will end up with 10 wins, which we would call false positives. So if you do a poor man’s calculation if you have 20 wins among those 100 experiments 50% of them would be false positives and only 50% real positives.
It’s a little bit more complicated because you also need to be aware of your Power levels (if they are not 100%) and when you clearly should have 10 real wins among those 20, this would mean you only had 90 experiments with no results which would lead to only 9 false positives and 11 true positives, but in that case you only had 89 experiments with no results etc.
To make it more easy for everyone we created a free calculator to calculate your own False Discovery Rate (FDR):
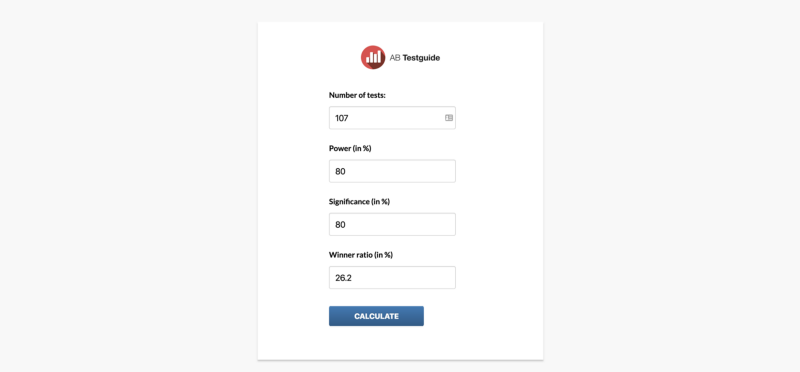
So to calculate your business case you would take every win, adjusted with the Type-M error and sum those combined results. This number is the number which you want to multiply with the True Discovery Rate (which is 100% minus FDR) to come to a real contribution for your Experimentation Revenue on short-term. Please do understand that 1 important variable is still discussable for the real attribution in this calculation: the length of impact. How long would your uplift last after implementation? 2 months? 10 years?
As a final note, I would like to mention that your optimization program will also lead to a whole bunch of user behaviour insights which can be used to make better business decisions in the future. This long term value is not part of this calculation. This calculation is also just focussing on optimizing an existing product (making more money) and not on, for instance, non-inferiority testing where you want to learn if a new feature is not costing you revenues. It’s also not focussing on user research like fake door testing to understand a potential product-market fit.
Related to this, what are your thoughts about running multiple experiments at once and how does this impact the contribution of Experimentation to revenue?
My initial answer is always: run more experiments. Even several separate A/B-experiments on the same population on the same page at the same time could be a good thing to do. Of course, you should calculate this. If the ROI of your experimentation program for optimization is still above the minimum threshold you are striving for then you should increase the number of experiments to grow faster, to outperform your competitors.
Overlapping experiments, in the end, will lead to more noise which leads to a lower ROI. It could be that one experiment is making you money, but the other one at the same time is lowering the impact of that winning one so it leads to a less big uplift or even a non-significant result. But in the end: would you rather run a 100 experiments program in 6 months with a 25% win percentage or a 200 experiments program with a 23% win percentage?
Let’s calculate that. Let’s say a win delivers you a $50,000 margin, and 1 A/B-test costs your company $2,500. In a mature experimentation company implementing a win will hardly cost you money (because every implementation is run as an experiment), but most companies are not there yet, so let’s say implementing a winner costs your company $5,000. To be able to calculate the False Discovery rate I assume a significance level of 90% and a power level of 80%. The Type-M error is already considered with the $50,000 margin per win.
Scenario A (100 experiments, 25 wins, FDR is then 31%):
- Costs: 100 * $2,500 + 25 * $5,000 = $375,000
- Extra margin: 25 * $50,000 * 69% = $862,500
- ROI: 230%
- Extra money made: $487,500
Scenario B (200 experiments, 46 wins, FDR is then 35%):
- Costs: 200 * $2,500 + 46 * $5,000 = $730,000
- Extra margin: 46 * $50,000 * 65% = $1,495,000
- ROI: 205%
- Extra money made: $765,000
To conclude?—?if your ROI limit to invest more money in experimentation is 200%, then even in scenario B you want to keep on investing money to run more experiments (which will lead to a little lower win percentage). The biggest difference of scenario B is the height and margin of the revenue growth. You are investing more money, with a lower ROI, but with a bigger absolute outcome. Of course, the ROI limit here is considering the risk of not getting to the result if the length of the impact of the winners is lower than considered upfront.
If you want to dive into more of this, I recently did a webinar presentation on how an analyst can add value to experimentation, which includes the business calculation topic, slides of that webinar can be downloaded as PDF. The slides also include some extra links to other calculators.
I think the only thing I’d add here is sometimes when you are working on product development, you really really need a clear signal on a test to understand whether a bigger investment should be made. In those cases, I feel, taking care to avoid unnecessary interactions is important. In other words, the more critical the learning?—?the less interaction you should have.
OK. Changing gears. Where do you find your inspiration for tests? And how do you prioritize them?
Our testing is based on hypotheses. These hypotheses are derived from continuous desk research, combining qualitative and quantitative user research insights, previous experiment outcomes, competitor analysis and scientific behavioural models and insights. Research is a really important part of Conversion Optimization. In my training and workshops on A/B-testing the research part always takes more time than the execution part of A/B-testing!
The hypotheses are prioritized on evidence. The more proof we have that a specific hypothesis could be true, the more we use it for creating A/B-tests to maximize revenue. If it’s starting to score less wins, it will be de-prioritized based on our scoring algorithm for prioritization.
Hypothesis with less proof are researched more to see if they could potentially be one of the next focus areas. After running an experimentation program for a while a hypothesis framework starts to appear. This is a consumer decision journey that can also be used as input for new product development and marketing campaigns.
As you hire for your agency, what are the traits that you look for?
It’s been a while since I hired someone myself. Nowadays the management team at Online Dialogue is responsible for most hires. But if I’m part of the hiring process for a specific job role I would first look for the right mindset, then see if there is a cultural fit and only then check if the experience/skills for the specific job are available or could become available fast.
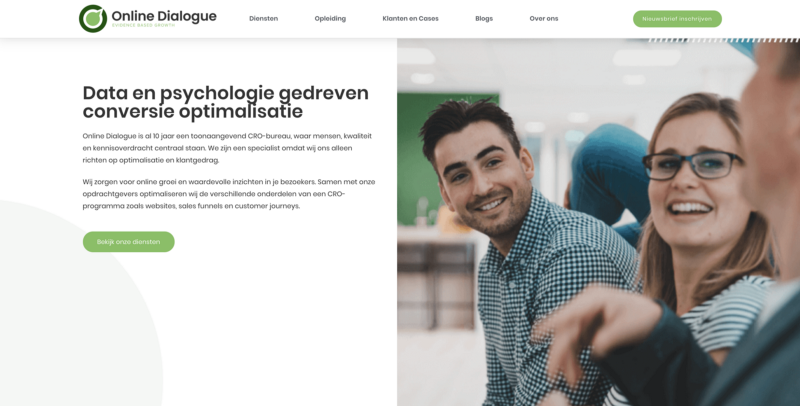
I’ve heard you think CRO will jobs die?—?what makes you say that?
I started using that in my presentations to wake up the people currently working in CRO. Most companies nowadays have a dedicated optimizer or even a whole dedicated team for optimization. They are people that made a difference in the company, that brought in money, that convinced upper management to spend more money on Conversion Optimization and Experimentation. They are proud, which makes total sense and which they should be.
But if you want to scale up to a culture of validation where every product and marketing team is making evidence-based decisions you will need a center of excellence (CoE). This validation center of excellence is responsible for a trustworthy process and the ease of use of tooling to support this. They are the ones that democratize validation throughout the whole organization.
Pride is not the right mindset for this CoE team. They should be humble. They are enablers that are there to let the other teams have their success. They should not judge and give those product and marketing teams time to fail. This is a different kind of DNA then currently available in the dedicated optimization team.
Once our market grows more mature the optimizers should become more mature too and take the next step to becoming an enabler. If they don’t, they will be out of a job someday. These current CRO jobs will also not be called CRO jobs anymore. It’s a daily routine enabled by the Center Of Excellence to support evidence-based growth.
If you want to dive into more of this, I recently recorded this center of excellence and CRO jobs will die presentation for an online summit. The slides of that presentation can also be downloaded. Let me know if you need me to wake up your company 🙂
These are awesome resources. Funny you should say that. Where I work, our Experimentation Center of Excellence definitely embodies the “enabler” role?—?where we partner with various Experimenters across the company to coach them on the benefits of an evidence-based approach, teaching them to be stronger Experimenters. We’re proudly humble 😉
Finally, it’s time for the Lightning Round!
How do you say Conversion Rate Optimization in Dutch?
“Conversie optimalisatie”. At least we dumped the “rate” part 🙂 would have been better if we would have dumped conversion too. In the end, it’s all about optimization.
How do you feel about the term “CRO” for this industry?
Evidence-Based Growth. Optimization, validation, experimentation. But the term is coined. I see it disappear in companies once they have a Center of Excellence for validation or just experimentation. The term itself will stick around though, just like SEO. Luckily CRO also means Chief Revenue Officer. Hopefully, the market will learn that CRO is not just running A/B-tests, that experimentation is more than just tweaking the conversion of existing products and that companies will grow to validation in their whole organization.
Describe Ton in 5 words or less.
Evidence-based growth enabler
Ton, thank you for joining the Conversation!
You are welcome! Keep on spreading the word.
Connect with Experimenters from around the world
We’ll highlight our latest members throughout our site, shout them out on LinkedIn, and for those who are interested, include them in an upcoming profile feature on our site.
Related posts:
- Don’t rely on macro goals with Gursimran (Simar) Gujral - July 26, 2024
- Navigating Experimentation at Startups with Avishek Basu Mallick - July 18, 2024
- People are the biggest challenge featuring Michael St Laurent - July 13, 2024